The AI revolution is moving at a breathtaking pace, with adoption rates that are surprising even the most seasoned technology executives. Recent industry data points to AI deployments accelerating much faster than cloud computing did in its early days – and that comparison is particularly striking given how transformative cloud computing has been over the past decade. It is that type of growth that is creating new challenges in cost management and optimization.
The Unprecedented Pace of AI Adoption
When Amazon CEO Andy Jassy recently noted that their AI business is growing three times faster than AWS did at a comparable stage, it highlighted just how extraordinary the current AI adoption curve truly is. This isn’t just big tech posturing – it reflects a fundamental shift happening across industries as organizations rush to harness AI’s potential.
According to McKinsey’s latest State of AI report (2024), AI adoption has seen a dramatic surge, with 72% of organizations now using AI in some capacity – a substantial increase from previous years where adoption hovered around 50%. Even more telling, 65% of organizations are already regularly using generative AI, and 67% expect to increase their AI investments over the next three years. This rapid adoption is creating both opportunities and challenges for enterprises.
The Hidden Cost Implications
While the transformative potential of AI is clear, what’s less obvious to many organizations is the significant cost implications of widespread AI deployment. Training and running large language models and other AI systems requires massive computing resources. For example:
- Training a single large language model can cost anywhere from $100,000 to several million dollars
- Ongoing inference costs for AI applications can quickly spiral into tens of thousands of dollars monthly
- Storage requirements for AI datasets and model versions create additional cost complexity
- Multi-cloud AI deployments introduce new layers of cost management challenges
This is where the critical intersection of AI adoption and FinOps becomes apparent. As organizations rapidly scale their AI initiatives, they need sophisticated approaches to manage and optimize the associated costs.
Why Traditional Cost Management Falls Short
Traditional IT cost management approaches simply aren’t equipped to handle the unique challenges of AI deployments:
- Dynamic Resource Usage: AI workloads can be highly variable, making static resource allocation inefficient
- Complex Dependencies: AI systems often span multiple services and cloud providers
- Rapid Innovation: New AI capabilities and services are constantly emerging, requiring flexible cost management
- Scale Challenges: As AI usage grows, costs can escalate exponentially without proper controls
The FinOps Imperative for AI Success
This is where modern FinOps solutions like DigitalEx and FinOps best practices become essential. FinOps provides the framework and tools needed to:
- Gain real-time visibility into AI-related cloud spend across all providers
- Implement automated cost optimization for AI workloads
- Align AI investments with business outcomes
- Enable proper chargeback and cost allocation for AI initiatives
- Forecast and budget for AI scaling
Best Practices for AI Cost Management
Organizations successfully managing their AI costs typically follow several key principles:
- Implement Comprehensive Monitoring – Deploy solutions that provide granular visibility into AI-related resource usage and costs across all cloud providers. This includes tracking compute, storage, and API usage specifically related to AI workloads.
- Optimize Early and Often – Don’t wait for costs to become problematic. Implement automated optimization strategies from the start, including:
- Right-sizing AI infrastructure
- Leveraging spot instances where appropriate
- Implementing automated scaling policies
- Optimizing model deployment strategies
- Enable Cross-functional Collaboration – Create processes that bring together:
- Data Science teams
- Engineering
- Finance
- Business stakeholders
This ensures all perspectives are considered in AI cost management decisions.
- Measure and Track ROI – Implement clear metrics to measure the business value generated by AI investments against their costs. This helps justify continued investment and identifies areas needing optimization.
Looking Ahead: The Growing Importance of AI FinOps
As AI adoption continues to accelerate, the need for sophisticated FinOps practices will only grow. Organizations that establish strong AI cost management foundations now will be better positioned to scale their AI initiatives sustainably.
Analysts are predicting that by 2026, organizations without formal FinOps practices will overspend on cloud by 40% or more. Given the higher cost intensity of AI workloads, the impact on AI initiatives could be even more significant.
Taking Action
Organizations looking to get ahead of this challenge should:
- Assess their current AI cost management capabilities
- Implement comprehensive cloud cost management solutions that support AI workloads
- Develop clear policies and processes for AI resource allocation and optimization
- Invest in training teams on FinOps practices specific to AI
- Regularly review and adjust their AI cost management strategy
The AI revolution is here, and it’s moving faster than anyone expected. Organizations that combine aggressive AI adoption with sophisticated FinOps practices will be best positioned to realize the full potential of AI while maintaining cost efficiency and control.
Success in the AI era requires more than just implementing the latest AI capabilities – it demands a thoughtful approach to managing the economics of AI at scale. As organizations continue to accelerate their AI initiatives, making FinOps a central part of their AI strategy will be crucial for sustainable success.
##
ABOUT THE AUTHOR
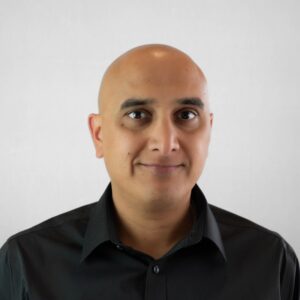
Sundeep Goel is the CEO of DigitalEx – a leader in the Cloud FinOps space with a particular focus on LLM workload optimization. He is an enterprise software veteran across several quality VC-backed technology companies and has held leadership roles at Netsuite, Adthena, and Procore. He has significant experience helping to scale up B2B software organizations commercially and has held CRO, COO, and VP Sales titles across his career.
Sundeep has a Bachelors degree in Computer Science from the University of Pennsylvania and currently lives in Austin, Texas with his wife, 2 boys, and puppy.